This article is a summary of a recent presentation by Jessica Bulthé, Data Science Business Partner at Mediahuis, shared at the WAN-IFRA Digital Media Europe event in Vienna. If you attended the event, you can download her presentation slideshere using the code sent via email by the organizers.
- A highly manual effort is required. And the more complex the subscription economy becomes, with ever more technologies to add to a publisher's tech stack, the more manual effort will be required
- It's a very slow way to find what is really working and what is not because you can't test all ideas at once. In a rapidly changing digital world of subscription economy you don't want to be slow in making changes
- The winner takes all in A/B testing, it leaves no room for personalization and differentiation. What works for young people might not work for older people, and visa versa. Are you willing to leave behind a group of people because they don't convert in the same way as the majority?
The solution: propensity modeling
Before propensity modeling, everyone is assumed to be just as likely to buy a subscription. Publishers employ a “one-size-fits-all”, spray-and-pray strategy that may well work for a small percentage of your audience but won't be optimal for maximizing the value of the majority of your readers.
Audience segmentation is indeed a step in the right direction. Targeting certain readers based on data points such as location, age or device type. But propensity modeling looks at the behavior of previous readers to then predict a new reader's likelihood to subscribe or churn.
The idea is that this model becomes more intelligent over time as it collects more data and learns from experience, being able to make predictions with increasing levels of accuracy.
How does Mediahuis recommend you get started with a propensity model?
- Collect data (1st party, behavioral, subscription status). Jessica noted that although they collect data for both anonymous and registered users, which has helped them build a large database, the model for registered readers does perform better and increases retention as deanonymization allows you to collect additional data points and assign this information to a single individual.
- Clean the data
- Identify patterns
- Make predictions for new users
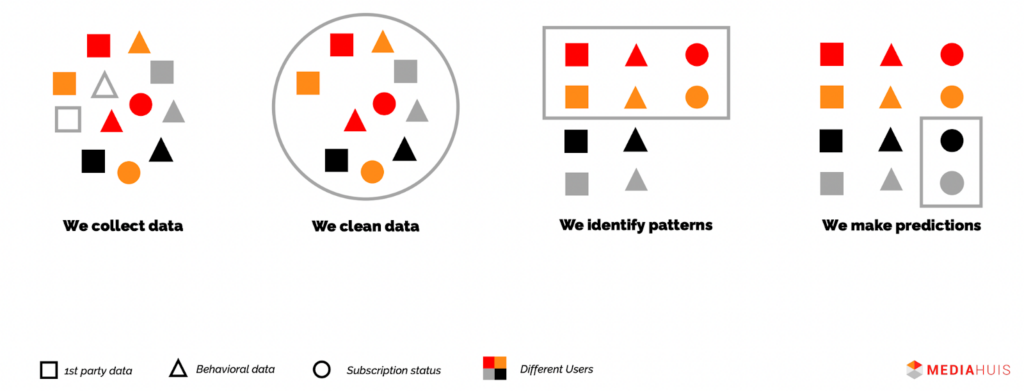
This can then be put into practice in your A/B tests, running a test on only one propensity group to optimize performance for that specific type of user:
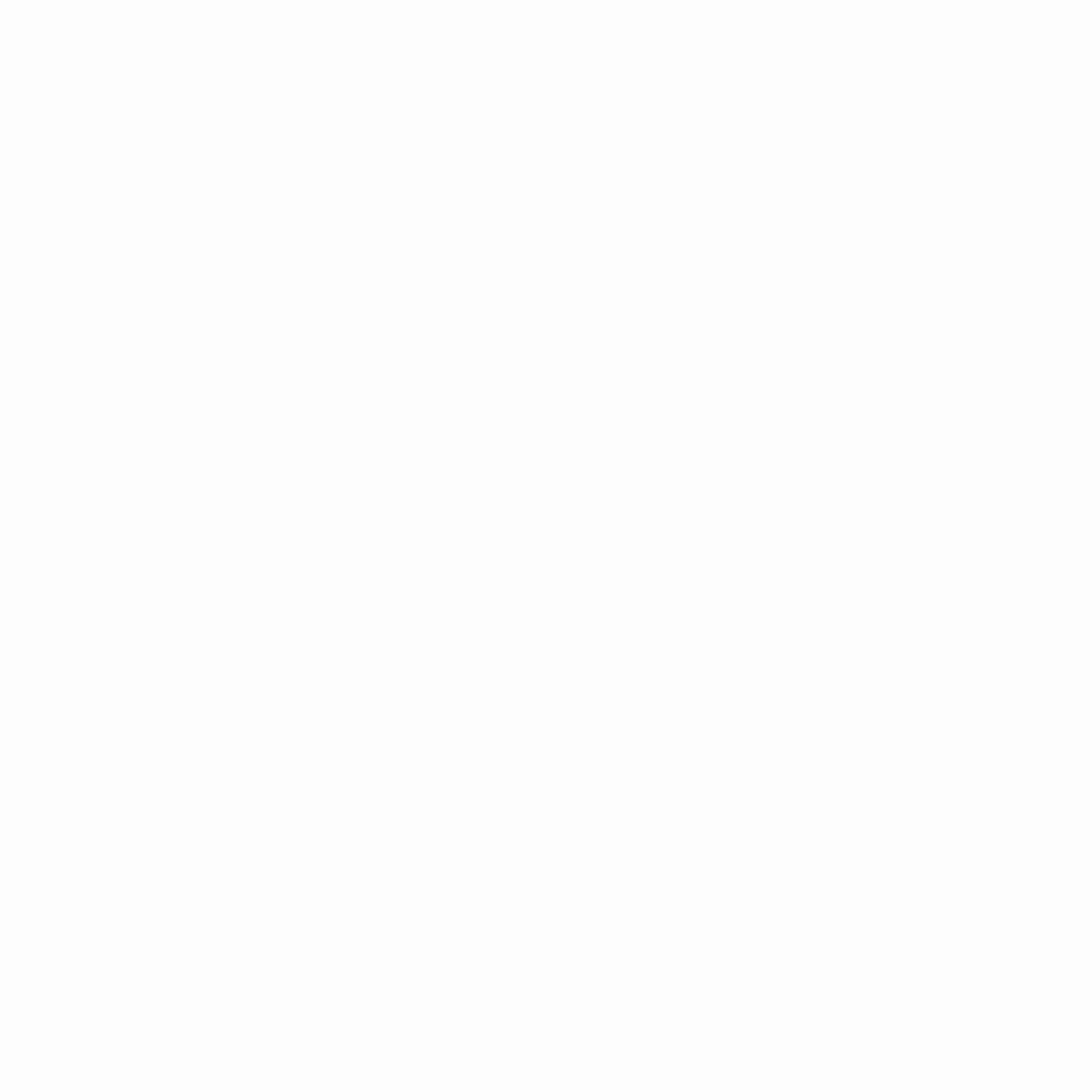
However, the key is not in having this model, but about making use of it in your daily work and customer journey. If the model still requires you to do a lot of manual work (such as A/B testing) you may well be data-informed, but you aren't automating the processes and are still limited in that you can't personalize customer experiences without having an army of marketers, sales, data analysts, etc.
> You'll also enjoy: 12 things you can learn from The Washington Post's strategy
From data-informed to data driven
Moving on from data-informed models that still require a lot of manual effort to data-driven with models that will do the testing for you and are incorporated into the entire customer journey to increase customer lifetime value.
Next-best-action modeling
Next-best-action modeling is a machine learning approach to generating user personalized communications and actions. It maps out the user journey and campaigns that a reader has been exposed to, linking it to 3-year ARPU. This information is then used to predict which actions and communications will best optimize the CLV of that micro-audience based on many possible variables, something that's too much for the human mind to do.
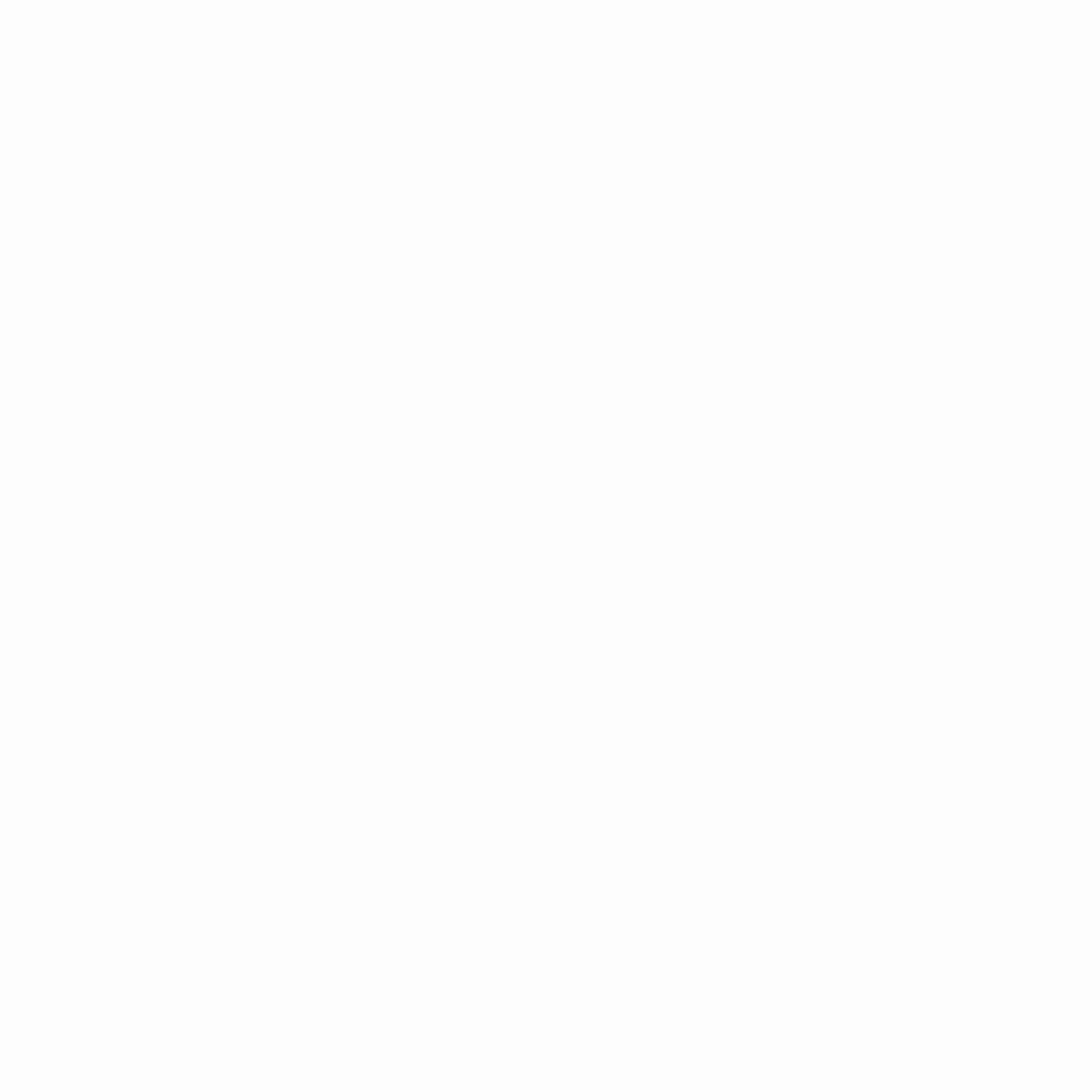
How do you put this model into practice?
“Start walking before you run!”
- Start by collecting and tidying all the data, implementing tools and putting the operations into place to continue these processes
- The propensity to buy model should come next
- Then the next-best-action model
How Mediahuis have used this model so far:
- A/B testing without models: To acquire first-party data points, discovering what helps retention
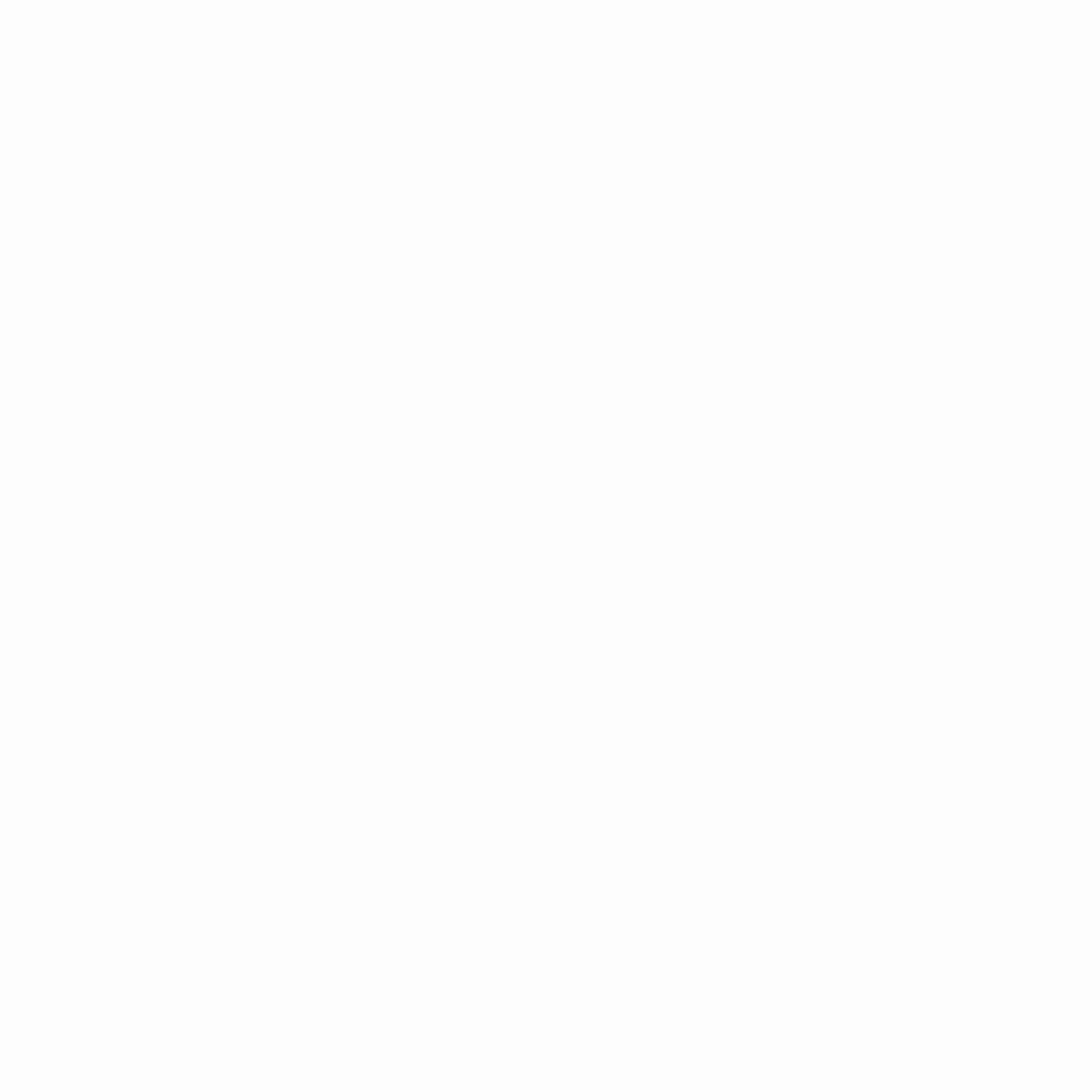
- Create a churn model: To differentiate between which customers have a higher potential churn risk. Do some more A/B testing to collect new data on what works for which types of customers.
For instance:
They gave “high propensity to churn” subscribers a call, increasing retention by 14.17%. This reduces time and resources by only calling subscribers who have the highest propensity to churn.
Those with a “medium propensity to churn” were sent a personalized email with a video from the editor-in-chief, sharing how subscription is valuable for democracy. This increased retention amongst these readers by 8.99%.
Whilst those with a “Low propensity to churn” were left alone, meaning the publisher saved time and resources.
- Next best action modeling. Once you collect enough data to know which campaigns/actions work well for which churn scores, you can start building your next-best-action modeling to automate the personalized customer journey.
The next step?
Mediahuis have now put their model into action to support acquisition. The next step is to automate personalized conversion and retention experiences. As Jessica rounded off with, “Automate all things normal, let the mind focus on change & creativity”/restricted-content]