Share This Article
After working as Head of Product in various large media groups, Olivier Martinez has now founded 255hex.ai to support companies in sourcing and implementing AI and generative AI tools.
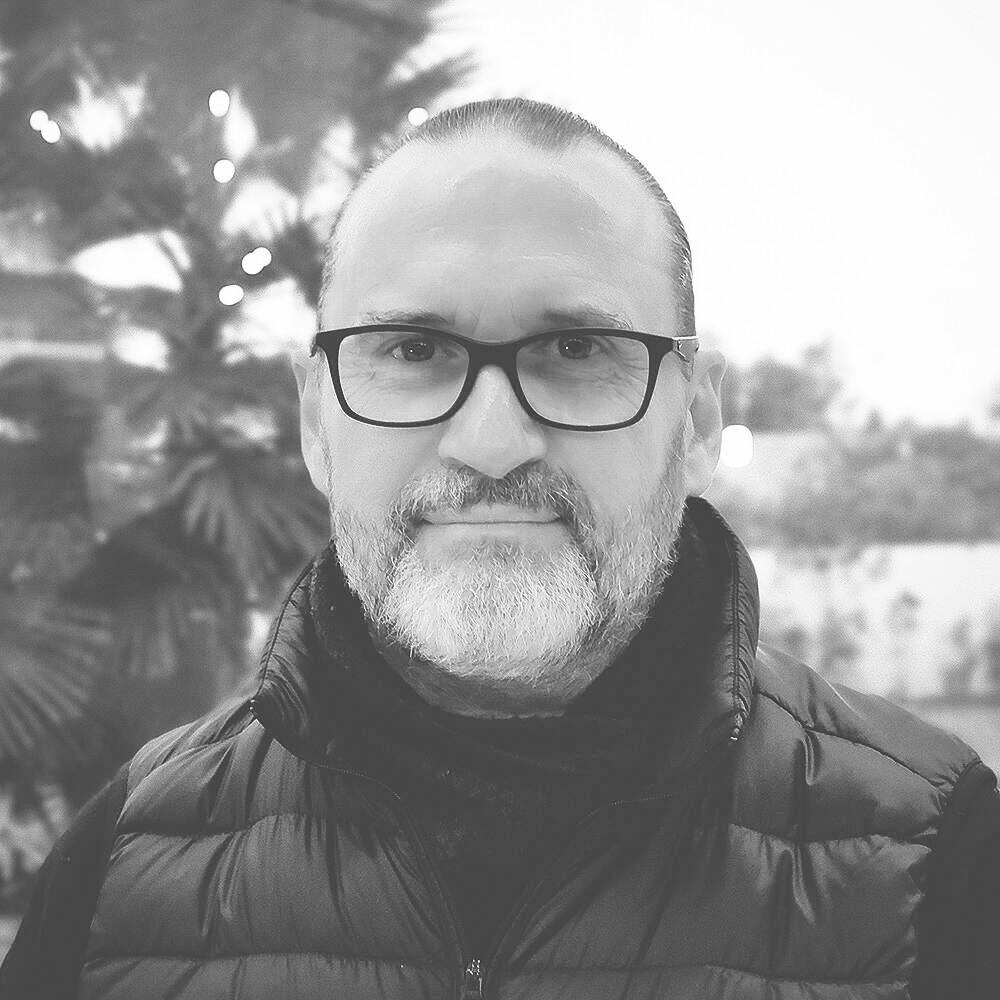
Artificial intelligence has been the talk of the town since late 2022 when ChatGPT, this dazzling phenomenon, gave us a lot to be excited about… “What if we used AI? The possibilities are endless, we'll definitely be able to profit from this!”
But wait a minute, what are we talking about exactly?
AI is a set of techniques and technologies that aim to imitate human cognitive processes, a significantly wider field than that of a conversational chatbot on steroids. And, within this much wider field, we're going to be looking to Machine Learning, because it's thanks to Machine Learning that we'll be able to learn to try to anticipate things. Such as, for example, the behavior of our readers.
Machine Learning is the basis of the current development of AI, including ChatGPT. It's such a fundamental concept that it's synonymous with AI in the minds of many. Machine learning can be summarized as a set of algorithms that allows a system to learn and improve automatically from its own experience without being explicitly programmed to do so. It's all a bit magical.
But, intelligence needs fuel. Data.
Importantly, however, these algorithms need fuel to function. And this fuel is data. Thanks to this data, algorithms can identify trends, patterns and relationships, allowing it to make decisions and probabilistic predictions. Yes, probabilities.
So data plays a more than crucial role in the performance and effectiveness of AI. WIthout data, these algorithms would be unable to learn, predict and adapt their behavior.
> You'll also enjoy: Beyond the human mind: Mediahuis propensity model
Data must be usable and relevant
But is “having data” enough? You already know the answer, and you're likely not happy about it. Nope, “having data” is not enough. It has to be available, relevant, of good quality and usable.
It sounds obvious, but the relevance of the data depends on the questions you want to solve. It's important to remember that even before choosing data, you need to understand the problem you want to solve and determine the objectives you want to assign to machine learning. This helps to identify the variables and features most relevant to the problem.
Example of this in action: propensity to subscribe to your paid product
To illustrate, let's take a random example of a publisher who wants to launch a paid offer and wonders if their loyal audience is ready to spend dollars on a subscription product.
The problem here is to predict whether a reader is likely to agree to pay a subscription.
The objectives assigned to Machine Learning could be to increase the subscription conversion rate, or even to adapt offers to the needs of individual readers.
We can therefore pre-select data that seems relevant to this use case:
- Engagement: number of articles read, frequency of visits, shared, etc.
- Content preferences: most read topics, authors, interests, etc.
- Demographics: age, gender, location, occupation, estimated income
- Temporality: time of day or day of the week when the reader consults the most content
But wait, where do they find this data? Hang on, they don't ask a reader to log in?! Without yet having launched a subscription offer, the only data they have is from analytics tools, meaning that the quality needed for AI isn't there. And this problem can't be solved with pixie dust…
So, before you even think about using artificial intelligence to predict reader behavior and optimize subscription conversion rates, you have to use your ‘human' intelligence to set up a logging system that will allow you to collect quality and exploitable data
Of course, this example is totally exaggerated and any resemblance to a real-life situation would be by chance…Since the launch of ChatGPT, nobody has ever tried to introduce AI without having first sorted the things needed to make it work…
Once your readers are logged in, and the data collected and structured, you'll have a host of use cases
For those of you who already have healthy and exploitable data, AI can be used in a whole host of ways. You could tackle your churn rate, for instance.
Data science allows us to analyze customer behavior in order to detect the risks of churn and determine typical profiles of “churners”. As for artificial intelligence, it allows us to create predictive models that take into account all data collected, automatically identifying all the possible causes of churn and segmenting users according to their level of loyalty. In concrete terms, the machine learns to recognize hidden user behavior patterns in the data to distinguish between the two possible types of users: churners and non churners.
HANAA GEMAYEL JABBOUR, Head of Marketing at L'Orient-Le Jour
Finally, and we may talk about this in more detail in future columns, whatever Machine Learning project you start, remember two things:
- You need data scientists, to do without them is to drive a 38 ton truck into a wall at high speed.
- Secondly, don't lose sight of the fact that an artificial intelligence project can never be sure of achieving a usable result in the time allotted, or even at all.