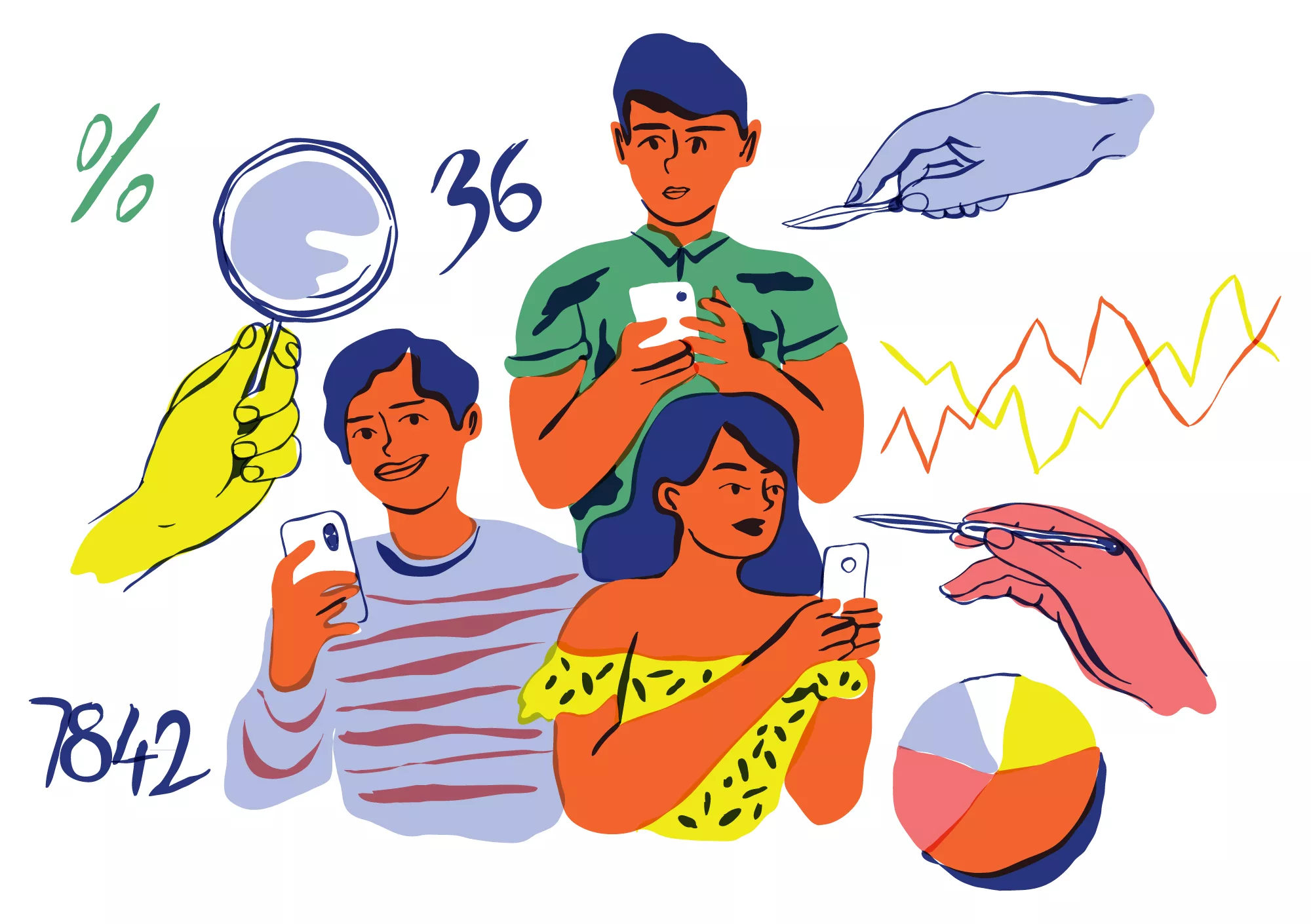
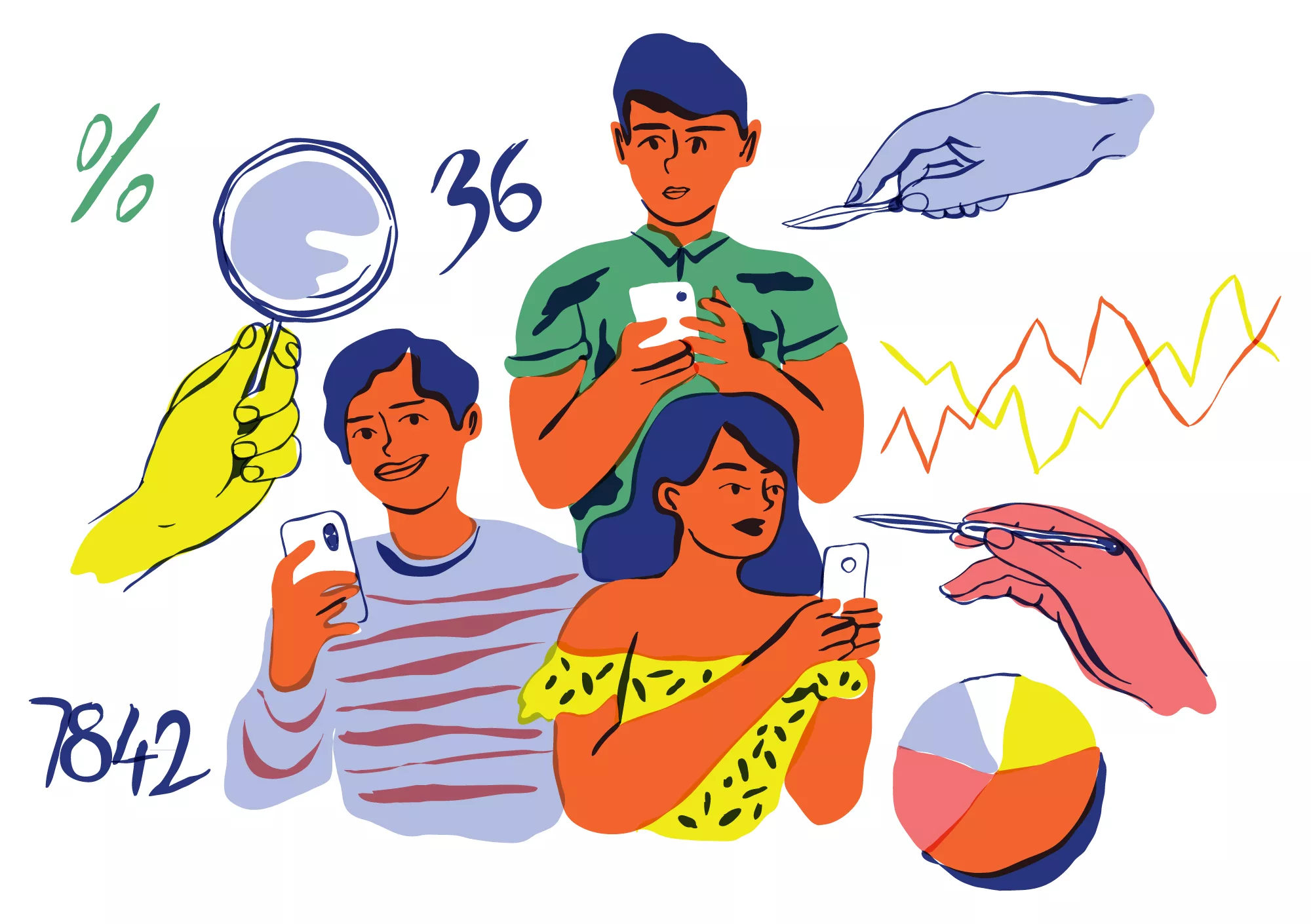
Today it’s all about data. I probably don’t need to explain to you how important it is for subscription success, but data is often still a specialist topic that many people are afraid of.
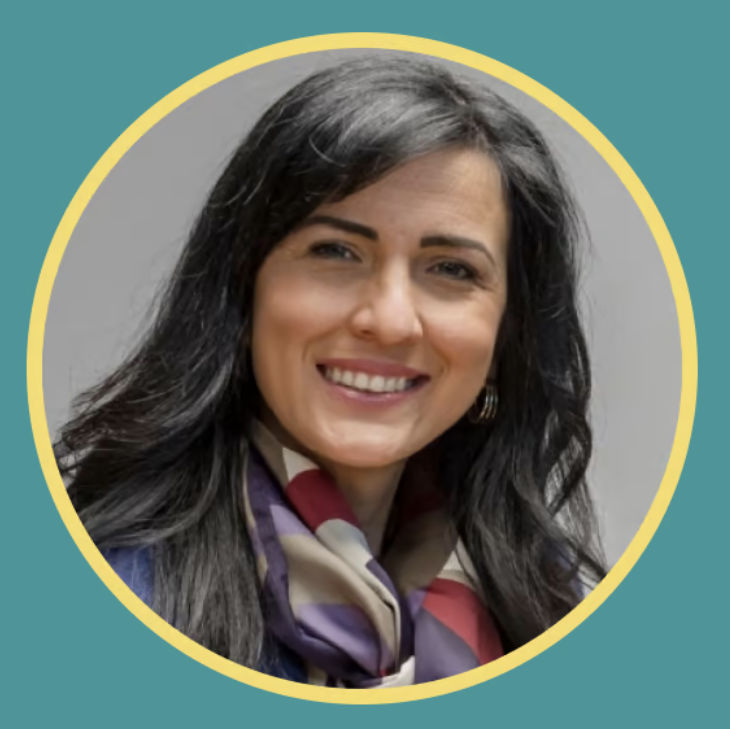
So that you can feel a little smarter in your next conversation with data colleagues, I’ve summarized three insights from an interview on my podcast, Subscribe Now, with data specialist Ana Moya (ex. Handelsblatt Media Group and Funke).
1. Find the right analytics method for your question
AI is being applied to many problems today, often as the default. However, this approach often doesn’t make sense. In many cases, a simpler analysis method—such as a basic hypothesis test or descriptive statistics—is sufficient. These traditional techniques can be more appropriate and effective for many analytical tasks.
Ana therefore recommends considering these four types of analysis for each question, considering what level of complexity is appropriate for the question:
- Descriptive statistics: What’s happening in my business?
- Exploratory statistics: Why is it happening?
- Predictive statistics: What’s likely to happen?
- Prescriptive statistics: What should I do now?
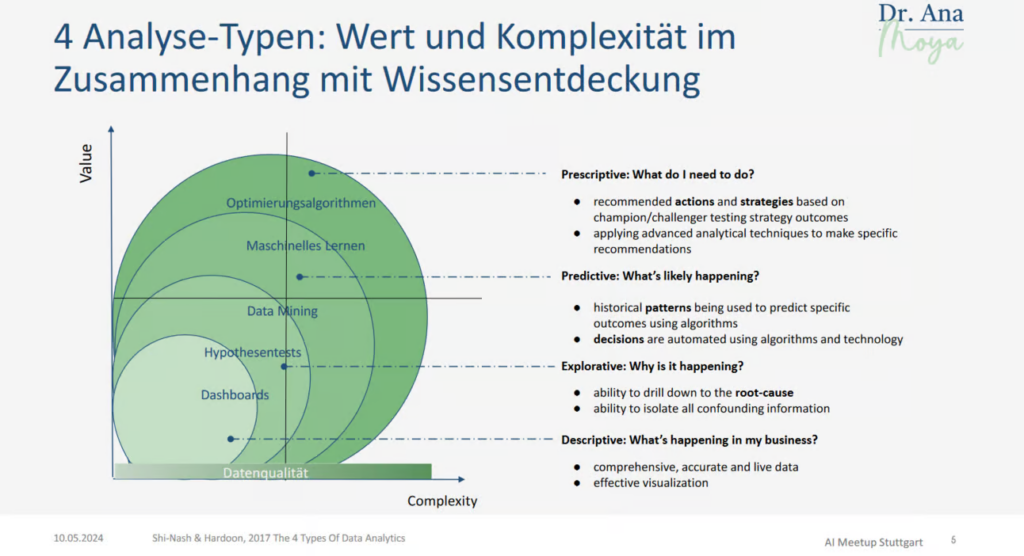
Important: All of these analyses only work if they are based on a solid data foundation. Otherwise, the old rule applies: Sh*t in, sh*t out!
2. To reduce churn, you need models that explain why users cancel
Many companies are currently introducing prediction scores to analyze which users are particularly likely to take out a subscription, accept a subscription upgrade, or cancel their subscription in the next X months.
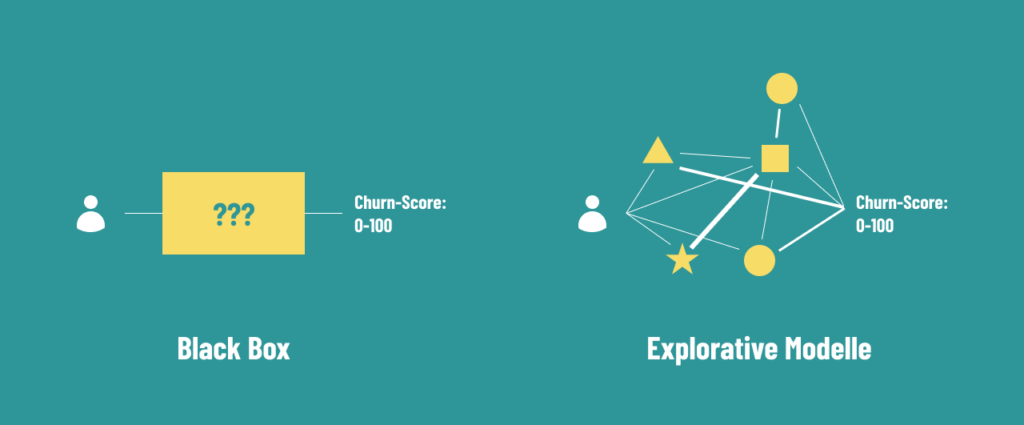
There are two ways to build these models:
- Pre-trained models that have already been optimized with subscription data and are often already integrated into tools, such as Piano
- Self-trained models that you develop in-house and feed with your own data
Although the latter is much more complex and requires a lot of know-how in the data science team, Ana recommends this approach.
She sees two advantages in this:
- You are tool-agnostic, so you can use these analyses independently of the systems used for subscription management and the like and combine data from different sources.
- These models provide a more precise understanding of how individual variables affect the probability of termination.
Pre-trained models are quicker to use and can often be implemented by laypeople, but they often remain a black box. That is, each user is assigned a value between 0 and 100, but it is not possible to understand exactly why.
If you work with such a black box, you can test a measure (e.g. an email campaign) and see whether it reduces the likelihood of cancellation.
However, it is better if you understand that, for example, payment by invoice has a major impact on the likelihood of cancellation. Then you can test measures to steer users to another payment method with a lower churn rate.
The success of this measure can then be measured in two steps:
- Were fewer subscriptions ordered on account?
- Did users in this test group cancel less often?
So you should try to improve the input variables rather than focusing on the output like a churn score.
A statistical model is particularly useful if it helps you understand the patterns behind the terminations, because then you can develop and prioritize targeted hypotheses and measures.
3. The difference between a CDP, a CRM, a data warehouse and a data lake
When it comes to data, you come across these terms all the time and it helps to understand the differences. to put it simply, they’re different ways of storing and structuring data.

- A data lake collects data from different sources in different formats. This includes data where it is not yet known whether and how it will be used and often the data is not yet finally structured and cleaned.
- In contrast, a data warehouse has a clearer structure. The data is (symbolically speaking) already stored in labeled boxes on pallets on a shelf in a warehouse. Imagine it like an Ikea warehouse, where you always know where to find the Billy shelf and how many of them are still in stock.
- A customer data platform (CPD) organizes the data around the customer. While the data warehouse contains all possible data, the CDP bundles different sources and all touchpoints with your users in order to understand exactly what a customer is interested in, what they last bought and when they last visited the website.
In addition, a CDP offers operational functions, such as personalization and campaign management, in order to implement marketing and communication measures based on data. - A customer relationship management (CRM) system manages the interactions between you and your customers. In contrast to the CDP, however, the CRM usually has a more limited view of interactions within certain systems and does not yet contain the data from all touchpoints.
Each of these systems has its advantages and disadvantages, so it always depends on the area of application what you need. However, it is important that the data is properly compared so that you have a single source of truth and no contradictory information that then leads to chaos.