This article is co-written by Katie Koschland and Liz Lohn.
AI is a divisive topic. Whatever your take is — from ‘it will kill us’ to ‘it will save us’ — there’s a tribe for that on the Internet.
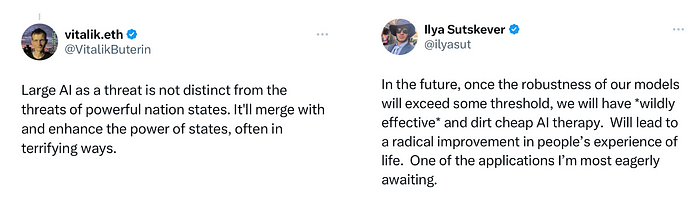
The stakes go up a level when we talk about AI in the news media. When it comes to generative AI, the public believes it might have a negative impact on news. At the same time, the level of comfort regarding news produced ‘with some help from AI’ is reasonably high.
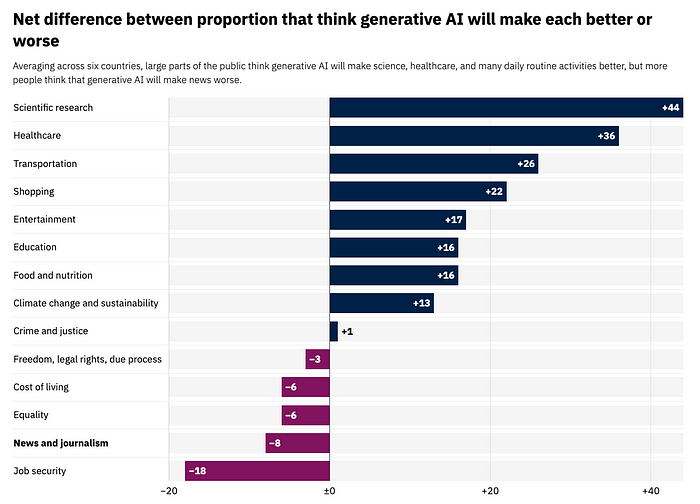
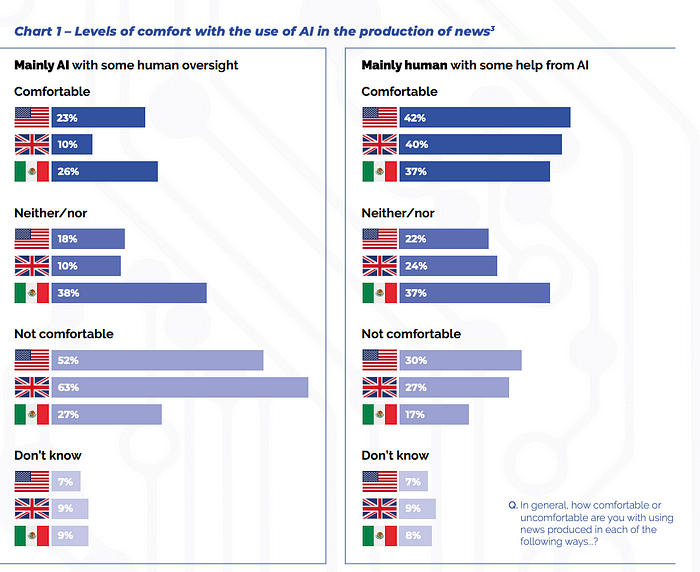
At the Financial Times, we believe that engaging with this space is crucial to address opportunities and threats emerging for media outlets. Through a lot of experimentation in 2024, we uncovered some promising generative AI use cases (a topic for a separate post) and leveraged predictive machine learning to support computational journalism in uncovering and developing stories that would otherwise stay untold.
Building a Scalable Solution
We’ve been privileged to lead a multidisciplinary team with the mission to bring focus to and accelerate the development of AI products at the Financial Times. One of our primary challenges lay in making data-driven reporting more scalable. In the FT newsroom, there were some very tech savvy data journalists who could do case by case data ingestion and analysis of complex data. But the process was often manual and isolated to specific stories or datasets. To overcome this, we needed solutions to enhance our journalists’ ability to mine data for insights consistently.
We launched an experiment focused on the UK Register of Parliament Members’ Interests — a complex, unstructured dataset listing MPs’ affiliations and financial interests. The important bits of information are often hidden there, obscured or presented as free text that is hard to run analysis on. By building a data pipeline, our team automated the extraction of key information, adding intelligent metadata and clustering entries for deeper insights.
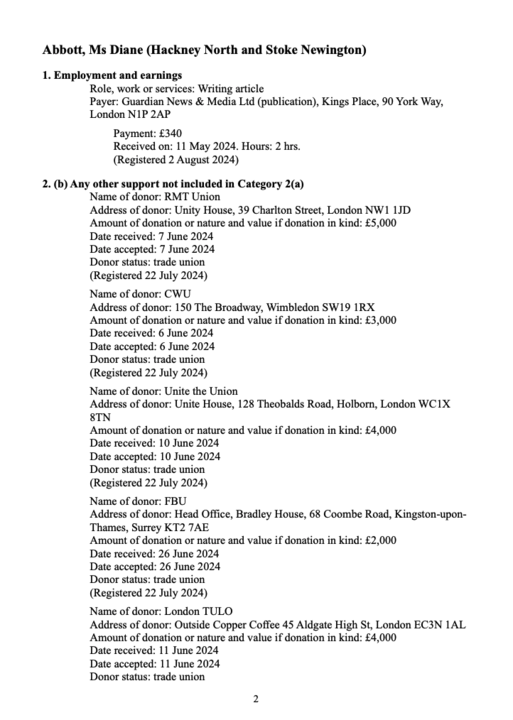
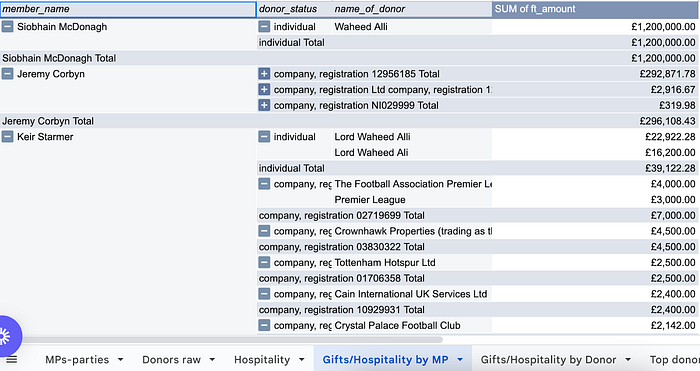
What it allows us to do in practice is for instance search one of the new UK government members and see their links to people and companies at a glance. Their interests can be aggregated into groups and entities that are not available in the original dataset. It also enables reverse querying of the data — asking questions like “Which MPs receive the most football tickets?” — as well as analysis of changes over time.
This capability allowed our journalists to quickly identify patterns and connections between UK government MPs and external entities, resulting in two published stories on MPs with previously hidden connections.
The first story raised critical questions about potential conflicts of interest as Labour advances its rental reform agenda. The second story revealed that Unite had shifted its financial support away from the central Labour party following Sir Keir Starmer’s move toward the centre, directing over £573,900 to individual left-leaning MPs. This analysis highlighted shifts in political and financial influence within the party.
By leveraging automated data pipeline paired with editorial analysis, the team was able to quickly and efficiently uncover these insights, accelerating the journalists’ ability to tell the story.
Expanding the Horizons
Having proven the hypothesis for ingesting and transforming a single dataset to unearth new stories, we are now expanding the capability to additional data sources and functionality. We’ve already replicated this success by adapting the model for U.S. data — starting with the Federal Election Commission records, which present a similar level of complexity and can benefit from a similar pipeline.
Additionally, we are developing a “fuzzy matching” capability, which would allow us to draw connections within and across datasets where exact matches might not exist. Fuzzy matching, or close proximity matching, helps identify subtle patterns by matching similar, though not identical, data points, such as variations in names or addresses. To implement this, we created a prototype pipeline that uses techniques like indel distance (measuring differences between text strings) and name normalisation processes. The initial testing has been promising and further refinement could generalise this tool for broader applications, enabling journalists to investigate a variety of datasets more efficiently.
Through this work, we’ve also identified several key challenges that need to be addressed in order to continue advancing data-driven journalism:
- Growing Data Complexity and Volume. As data complexity and volume increase, our ability to maintain a competitive edge in storytelling requires more advanced capabilities.
- Varied, Inconsistent and Unstructured Data. Journalists often face difficulties working with unstructured and inconsistent data sets. This is particularly challenging when working at scale and with multiple data sources, and when very few data sources have broad utility across various reporters’ subject-matter specialisms.
- Time-Consuming Data Acquisition. Obtaining large datasets can be a labour-intensive process, which slows down the newsroom’s ability to discover insights more rapidly. Often, as in the case of the Register stories, it takes far longer to simply get the data than to analyse it enough to tell the story.
- Difficulty in Connecting Insights. Discrepancies across various data sources make it harder to rapidly link key insights, potentially leaving important gaps in our stories and limiting our ability to uncover hidden narratives.
Looking into the future, we see three main opportunities areas to further enhance story finding in the FT newsroom:
- Information Extraction & Insight Generation. Using AI to extract names of individuals and organisations mentioned in unstructured data, group related data, and conduct connection analysis to uncover trends and patterns in large datasets that might otherwise go unnoticed.
- Signal-Based Notifications. Developing services that alert journalists when new, relevant signals emerge from data sources, ensuring early detection of important events or trends.
- Automatic Data Processing. Building pipelines for the automatic ingestion, cleaning, and parsing of unstructured data sources to establish a strong data foundation for sophisticated, exclusive analysis other news organisations will struggle to match.
Conclusion
Our experiments have highlighted the transformative potential of automated robust data pipelines and AI-driven analysis in story finding. Data pipelines uncover hidden narratives within complex datasets, while AI equips journalists with advanced tools to perform more sophisticated analyses, elevating their story finding capabilities.
We have seen the potential of AI-driven discovery while identifying challenges, such as managing growing data complexity and volume. As we refine these tools, we remain committed to responsible AI adoption, helping journalists tell richer, data-driven stories that reinforce the FT’s commitment to rigorous, impactful journalism.
Credits
The multidisciplinary team working on these capabilities includes: Chris Cook, Martin Stabe, Oliver Hawkins, David Djambazov, Ivan Nikolov, Zdravko Hvarlingov, Laura Paul, Jorge Sanchez-Cano, Desislava Vasileva, Erin O’Handley, Chris Mears, Liz Kessick.